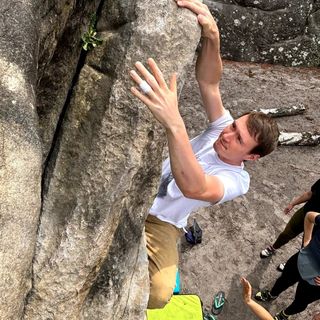
Publications
Publications
-
Dewolf, N. (2024). A comparative study of conformal prediction methods for valid uncertainty quantification in machine learning (Doctoral dissertation). Ghent University. http://hdl.handle.net/1854/LU-01HVK8CNHPMDFGQ8BGF86VMVTY
(Note: The Arxiv version can be found here.) -
Vancraeynest-De Cuiper, B., Bridgeman, J., Dewolf, N., Haegeman, J. & Verstraete, F. (2023). One-dimensional symmetric phases protected by frieze symmetries. Phys. Rev. B. https://link.aps.org/doi/10.1103/PhysRevB.107.115123
-
Dewolf, N., Baets, B.D. & Waegeman, W. (2023). Valid prediction intervals for regression problems. Artif Intell Rev. https://doi.org/10.1007/s10462-022-10178-5
(Note: Due to a small typesetting error1, the second author appears as “Baets, B.D.” while it should be “De Baets, B.”) -
Dewolf, N. (2019). Spatial Symmetries and Symmetry Breaking with Matrix Product States. (Master’s thesis). Ghent University. https://lib.ugent.be/en/catalog/rug01:002782900
Preprints:
- Dewolf, N., De Baets, B. & Waegeman, W. (2023). Conditional validity of heteroskedastic conformal regression. https://arxiv.org/abs/2309.08313
Conferences
- Dewolf, N., De Baets, B., and Waegeman, W. (2023). Conditional Conformal Prediction. Workshop on Uncertainty in Machine Learning (WUML).
- Dewolf, N., De Baets, B., and Waegeman, W. (2022). Valid prediction intervals for regression problems. Joint International Scientific Conferences on AI and Machine Learning (BNAIC/BeNeLearn).
- Dewolf, N., De Baets, B., and Waegeman, W. (2021). Regression problems in machine learning. Data Science, Statistics & Visualisation (DSSV) and the European Conference on Data Analysis (ECDA).
- Dewolf, N., De Baets, B., and Waegeman, W. (2021). Calibrated prediction intervals. Workshop on Uncertainty in
Machine Learning (WUML).
-
The publisher was made aware of this issue before publication, but did not take our comment into account. ↩